Underwriting Takeaways for Insurtechs
One of the most critical areas where technology is making a substantial impact is underwriting. Underwriting, the process of evaluating risk and determining policy terms.
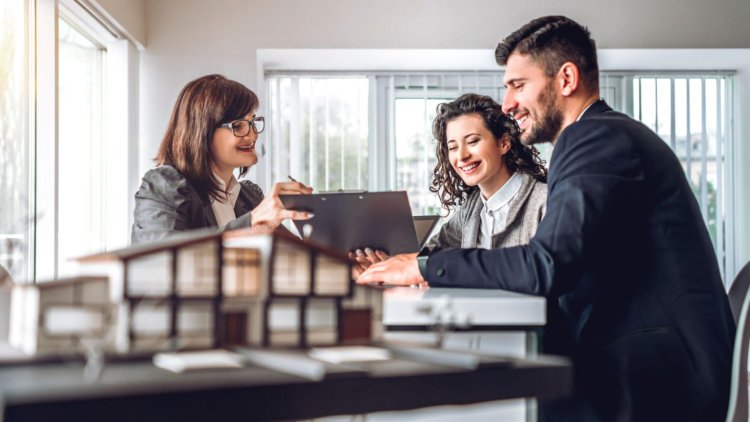
The insurance industry is undergoing a significant transformation with the advent of technology. Insurtechs—startups and companies leveraging technology to innovate and improve the insurance experience—are at the forefront of this change. One of the most critical areas where technology is making a substantial impact is underwriting. Underwriting, the process of evaluating risk and determining policy terms, is essential for the profitability and sustainability of insurance companies. For insurtechs, mastering underwriting is crucial to their success. This article explores key underwriting takeaways for insurtechs, providing insights into how technology can enhance this fundamental process.
Understanding the Evolution of Underwriting
Underwriting has traditionally been a labor-intensive process involving manual data collection, risk assessment, and decision-making. However, the rise of insurtechs is reshaping this landscape. Technology-driven underwriting leverages big data, artificial intelligence (AI), and machine learning (ML) to streamline and enhance the process. This evolution offers several advantages:
- Efficiency Gains: Automation reduces the time required to process applications and assess risks, allowing for quicker decision-making.
- Accuracy Improvement: Advanced algorithms and data analytics improve risk assessment accuracy, leading to more precise underwriting decisions.
- Cost Reduction: By automating routine tasks, insurtechs can lower operational costs associated with underwriting.
Key Technologies Transforming Underwriting
1. Big Data Analytics
Big data analytics is a cornerstone of modern underwriting. Insurtechs use large datasets to gain insights into risk factors, customer behavior, and market trends. By analyzing historical data, companies can identify patterns and predict future risks with greater accuracy. This data-driven approach enables:
- Risk Prediction: More accurate predictions of potential risks based on comprehensive data analysis.
- Customer Segmentation: Better segmentation of customers based on their risk profiles, leading to personalized policy offerings.
2. Artificial Intelligence (AI)
AI technologies are revolutionizing underwriting by enhancing decision-making processes. AI-powered systems can analyze vast amounts of data quickly and identify complex patterns that human underwriters might miss. Key applications include:
- Automated Risk Assessment: AI algorithms assess risks in real-time, providing immediate feedback and recommendations.
- Fraud Detection: AI systems can detect anomalies and patterns indicative of fraudulent activities, reducing the risk of fraudulent claims.
3. Machine Learning (ML)
Machine learning models improve underwriting accuracy by learning from historical data and continuously adapting to new information. ML applications in underwriting include:
- Predictive Modeling: ML models predict the likelihood of claims based on various factors, helping insurers set appropriate premiums.
- Dynamic Pricing: ML enables dynamic pricing strategies by adjusting premiums based on real-time data and risk assessments.
Best Practices for Insurtech Underwriting
1. Embrace Data-Driven Decision Making
Insurtechs should prioritize data-driven decision-making in their underwriting processes. Leveraging big data and advanced analytics allows for more informed risk assessments and policy pricing. Best practices include:
- Data Integration: Integrate diverse data sources, including customer data, market trends, and historical claims data, for a comprehensive risk assessment.
- Real-Time Analytics: Implement real-time analytics to make timely and accurate underwriting decisions.
2. Implement Robust Fraud Detection Mechanisms
Fraud prevention is a critical aspect of underwriting. Insurtechs should incorporate robust fraud detection mechanisms to safeguard against fraudulent activities. Key strategies include:
- AI and ML Integration: Use AI and ML algorithms to identify and flag potential fraudulent claims based on historical patterns and anomalies.
- Continuous Monitoring: Continuously monitor transactions and claims for suspicious activities to detect and prevent fraud proactively.
3. Foster Collaboration Between Technology and Human Expertise
While technology plays a crucial role in modern underwriting, human expertise remains valuable. Insurtechs should aim to create a synergy between technology and human underwriters. Best practices include:
- Hybrid Approaches: Combine AI and ML insights with human judgment to make well-rounded underwriting decisions.
- Training and Development: Invest in training programs to equip underwriters with the skills needed to work effectively with advanced technologies.
Challenges and Solutions in Insurtech Underwriting
1. Data Privacy and Security
Handling sensitive customer data poses privacy and security challenges. Insurtechs must ensure that their underwriting processes comply with data protection regulations and implement robust security measures. Solutions include:
- Compliance: Adhere to data protection regulations such as GDPR and CCPA to ensure customer data privacy.
- Cybersecurity Measures: Implement advanced cybersecurity protocols to protect against data breaches and unauthorized access.
2. Integration with Legacy Systems
Integrating new technologies with existing legacy systems can be challenging. Insurtechs should focus on seamless integration to maximize the benefits of technology. Solutions include:
- API Development: Develop APIs to facilitate smooth integration between new technologies and legacy systems.
- Phased Implementation: Implement new technologies in phases to minimize disruptions and ensure compatibility with existing systems.
3. Balancing Automation with Human Oversight
While automation enhances efficiency, it is essential to balance it with human oversight to maintain quality and accuracy. Solutions include:
- Human-in-the-Loop: Incorporate human oversight in automated processes to ensure accuracy and address complex cases.
- Regular Audits: Conduct regular audits of automated systems to identify and rectify any issues or inaccuracies.
Future Trends in Insurtech Underwriting
1. Increased Use of Predictive Analytics
Predictive analytics will become more prevalent in underwriting, allowing insurtechs to anticipate and mitigate risks more effectively. Future trends include:
- Enhanced Risk Models: Development of more sophisticated risk models using advanced analytics and machine learning techniques.
- Proactive Risk Management: Utilization of predictive insights to proactively manage and mitigate risks before they materialize.
2. Growth of Personalized Insurance Products
Personalization will continue to drive innovation in underwriting. Insurtechs will offer increasingly tailored insurance products based on individual risk profiles and preferences. Trends include:
- Customized Policies: Development of highly customized insurance policies that cater to specific customer needs and preferences.
- Behavior-Based Pricing: Introduction of behavior-based pricing models that adjust premiums based on individual behavior and risk factors.
3. Expansion of Blockchain Technology
Blockchain technology will play a significant role in underwriting by enhancing transparency and security. Future applications include:
- Smart Contracts: Use of smart contracts to automate and streamline underwriting processes, reducing administrative overhead and errors.
- Data Integrity: Leveraging blockchain for secure and tamper-proof data storage, ensuring the integrity of underwriting information.
Underwriting is a critical component of the insurance industry, and insurtechs are redefining this process through technology. By leveraging big data, artificial intelligence, and machine learning, insurtechs can enhance risk assessment accuracy, improve efficiency, and reduce costs. Embracing data-driven decision-making, implementing robust fraud detection mechanisms, and fostering collaboration between technology and human expertise are key best practices for insurtech underwriting. Addressing challenges such as data privacy, legacy system integration, and balancing automation with human oversight is essential for successful underwriting transformation.
1. What is underwriting in the context of insurance, and why is it important?
Underwriting is the process by which insurers evaluate the risks associated with providing coverage to applicants. This involves assessing factors such as health, financial stability, and other relevant information to determine the terms of the insurance policy and the premium amount. It is crucial because it ensures that the insurer can manage risk effectively, set appropriate premiums, and maintain profitability.
2. How has technology changed the traditional underwriting process?
Technology has revolutionized underwriting by introducing automation, big data analytics, AI, and machine learning. These technologies streamline data collection, risk assessment, and decision-making processes, leading to faster processing times, improved accuracy, and cost reduction. Automation handles routine tasks, while AI and machine learning provide deeper insights into risk factors and fraud detection.
3. What role does big data analytics play in modern underwriting?
Big data analytics plays a critical role in modern underwriting by providing insurers with comprehensive insights from large datasets. It helps identify patterns and trends that improve risk assessment accuracy and customer segmentation. By analyzing historical claims, market trends, and customer behavior, insurers can make more informed decisions and offer personalized insurance products.
4. How does artificial intelligence (AI) enhance the underwriting process?
AI enhances underwriting by automating risk assessments and providing real-time feedback. AI algorithms analyze vast amounts of data to identify patterns and anomalies that human underwriters might miss. This leads to more accurate risk evaluations, faster decision-making, and improved fraud detection.
5. In what ways does machine learning (ML) improve underwriting accuracy?
Machine learning improves underwriting accuracy by continuously learning from historical data and adapting to new information. ML models predict the likelihood of claims and adjust premiums based on real-time data. This dynamic approach allows for more precise risk assessments and personalized policy pricing.
6. What are some best practices for insurtechs to implement data-driven decision-making in underwriting?
Insurtechs should integrate diverse data sources, including customer data, market trends, and historical claims, to enable comprehensive risk assessments. Implementing real-time analytics is also essential for timely decision-making. Investing in data management systems and ensuring data quality are crucial for effective data-driven underwriting.
7. How can insurtechs effectively prevent fraud in underwriting?
Insurtechs can prevent fraud by integrating AI and ML algorithms that detect unusual patterns and anomalies indicative of fraudulent activities. Implementing continuous monitoring systems and conducting regular audits of claims and transactions can further enhance fraud detection and prevention efforts.
8. Why is it important for insurtechs to balance automation with human oversight in underwriting?
Balancing automation with human oversight is important to ensure accuracy and quality in underwriting. While automation handles routine tasks and processes large volumes of data, human expertise is essential for addressing complex cases and making nuanced decisions. A hybrid approach ensures that automated systems are effectively guided by human judgment.
9. What are the main challenges associated with data privacy and security in underwriting, and how can they be addressed?
Challenges include protecting sensitive customer data from breaches and ensuring compliance with data protection regulations such as GDPR and CCPA. Insurtechs can address these challenges by implementing robust cybersecurity measures, encryption, and secure data storage solutions. Regular audits and compliance checks are also crucial.
10. How can insurtechs integrate new technologies with existing legacy systems?
Integration challenges can be addressed by developing APIs that facilitate communication between new technologies and legacy systems. A phased implementation approach allows for gradual integration, minimizing disruptions. Additionally, collaborating with technology partners and investing in middleware solutions can ease the integration process.
11. What role does real-time analytics play in the underwriting process?
Real-time analytics enables insurers to make immediate and informed decisions by analyzing data as it becomes available. This approach allows for faster risk assessments, dynamic pricing adjustments, and timely identification of potential issues or opportunities, enhancing overall underwriting efficiency.
12. How can predictive analytics be used to improve underwriting accuracy?
Predictive analytics uses historical data and statistical algorithms to forecast future risks and outcomes. By analyzing patterns and trends, predictive models can anticipate the likelihood of claims and adjust underwriting decisions accordingly. This helps insurers set more accurate premiums and identify high-risk applicants.
13. What are some examples of personalized insurance products that can be developed using technology?
Examples include usage-based insurance (UBI) for auto coverage, where premiums are based on driving behavior; telematics-based health insurance that adjusts premiums based on physical activity; and customized property insurance policies that consider specific risks associated with individual properties. Technology enables insurers to tailor products to individual needs and preferences.
14. How does blockchain technology contribute to underwriting processes?
Blockchain technology enhances underwriting by providing secure, transparent, and tamper-proof data storage. Smart contracts automate underwriting processes and ensure that policy terms are executed accurately. Blockchain also improves data integrity and reduces the risk of fraud by creating an immutable record of transactions.
15. What are the potential benefits of adopting machine learning in underwriting?
Machine learning offers benefits such as improved accuracy in risk prediction, dynamic pricing, and efficient claims processing. ML algorithms can analyze vast amounts of data to identify patterns and trends, leading to more precise underwriting decisions and better risk management.
16. How can insurtechs ensure the accuracy of AI and ML models used in underwriting?
Ensuring accuracy involves training models with high-quality, relevant data and regularly updating them based on new information. Continuous monitoring and validation of model performance are crucial to identify and address any issues. Collaborating with data scientists and domain experts can also enhance model accuracy.
17. What strategies can insurtechs use to address integration issues with legacy systems?
Strategies include using middleware solutions to bridge gaps between new technologies and legacy systems, adopting API-based integration for seamless data exchange, and conducting thorough testing before full-scale implementation. Gradual integration and stakeholder involvement are also key to overcoming integration challenges.
What's Your Reaction?
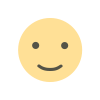
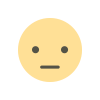
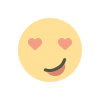
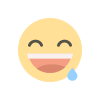
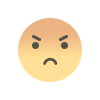
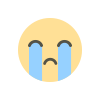
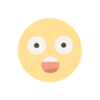